Artificial Intelligence and Machine Learning: An In-Depth Exploration
**SEO Summary: Artificial Intelligence and Machine Learning: An In-Depth Exploration** This comprehensive exploration of Artificial Intelligence (AI) and Machine Learning (ML delves into the foundational concepts, methodologies, and applications of these transformative technologies. The article outlines the distinctions between narrow and general AI, alongside a detailed discussion of ML types, including supervised, unsupervised, and reinforcement learning. Real-world applications of AI and ML across various sectors, such as healthcare,, and transportation, are highlighted, demonstrating their potential to revolutionize industries. Additionally, the essay addresses critical ethical considerations, including privacy, bias, and accountability, that accompany the adoption of AI technologies. As the future of AI and ML unfolds, the essay emphasizes the importance of responsible implementation and workforce readiness. Engaging with these technologies can unlock significant opportunities while addressing the challenges they present.
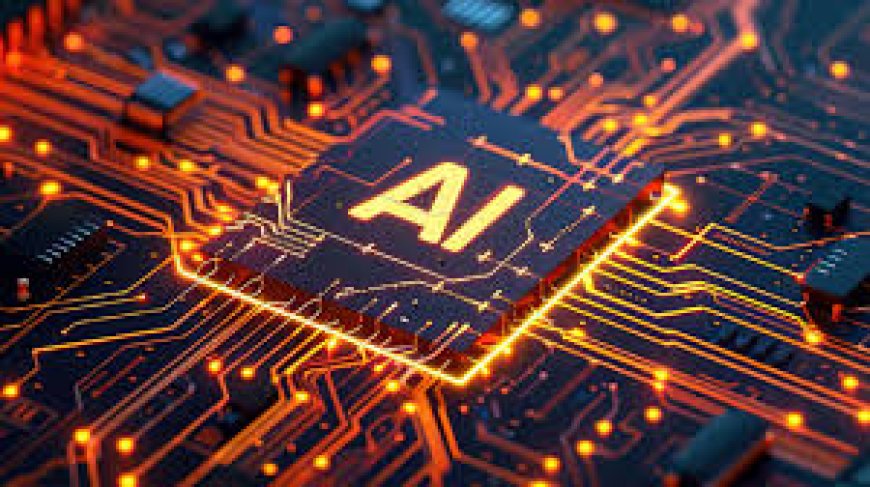
Introduction
The rapid advancement of technology in the 21st century has led to the emergence of artificial intelligence (AI) and machine learning (ML) as pivotal fields in computer science and related disciplines. AI refers to the simulation of human intelligence in machines that are programmed to think and learn like humans, while ML is a subset of AI that focuses on the development of algorithms that enable computers to learn from and make predictions based on data. This essay explores the foundations of AI and ML, their applications across various sectors, the ethical considerations they present, and future challenges and opportunities.
At the core of AI and ML lies the ability to process vast amounts of data and extract meaningful insights. The algorithms that underpin these technologies have evolved significantly, driven by innovations in computational power and data availability. Techniques such as neural networks, deep learning, and natural language processing have revolutionized how machines interpret complex information, enabling them to perform tasks previously thought to require human intuition and creativity.
The applications of AI and ML are multifaceted and span numerous sectors. In healthcare, for instance, AI systems assist in diagnosing diseases with remarkable accuracy, analyzing medical images, and personalizing treatment plans. In finance, ML algorithms detect fraudulent transactions in real-time, optimizing risk management and enhancing security measures. The retail industry has also embraced these technologies, utilizing predictive analytics to tailor marketing strategies and improve customer experiences.
However, the rise of AI and ML is not without its ethical considerations. Issues such as data privacy, algorithmic bias, and the potential for job displacement raise critical questions about the responsible deployment of these technologies. As AI systems become more integrated into daily life, it is essential to establish frameworks that ensure transparency, accountability, and fairness in their operations.
Looking ahead, the future of AI and ML presents both challenges and opportunities. As these technologies continue to evolve, they hold the potential to address pressing global issues, such as climate change and resource management. Yet, fostering public trust and ensuring equitable access to the benefits of AI will require collaborative efforts among technologists, policymakers, and society at large.
Foundations of Artificial Intelligence and Machine Learning
Artificial intelligence can be broadly categorized into two types: narrow AI and general AI. Narrow AI is designed to perform specific tasks, such as playing chess or driving a car, whereas general AI, which remains largely theoretical, would possess the ability to perform any intellectual task that a human can do. The development of AI involves multiple domains, including natural language processing, computer vision, and robotics, each contributing to the overall functionality of intelligent systems.
Machine learning (ML), in contrast, relies on the analysis of large datasets to allow machines to identify patterns and make decisions without being explicitly programmed for every scenario. ML algorithms are generally classified into three types: supervised learning, unsupervised learning, and reinforcement learning. Supervised learning involves training a model on labeled data, where the input-output pairs guide the learning process. This approach is widely used in applications such as image recognition and spam detection, where the model learns to associate specific features with desired outcomes.
Unsupervised learning, on the other hand, uncovers hidden patterns in unlabeled data, making it particularly useful for clustering and anomaly detection. Techniques such as k-means clustering and hierarchical clustering enable organizations to segment customers based on purchasing behavior or identify unusual patterns in network traffic, which can be critical for fraud detection and cybersecurity.
Reinforcement learning represents a unique paradigm where agents learn to make decisions through trial and error within a dynamic environment. This type of learning mimics human learning processes, allowing AI to adapt and improve over time. Applications of reinforcement learning are evident in robotics, where autonomous agents learn to navigate complex environments, and in gaming, where AI systems have achieved superhuman performance in games like Go and Dota 2.
As AI and ML technologies continue to evolve, the ethical implications and societal impacts of these innovations require careful consideration. Issues such as data privacy, algorithmic bias, and the potential for job displacement must be addressed to ensure that the benefits of AI are distributed equitably. Furthermore, interdisciplinary collaboration among computer scientists, ethicists, and policymakers will be essential in shaping a future where AI serves humanity positively.
Applications of Artificial Intelligence and Machine Learning
The applications of AI and ML are diverse and expanding rapidly, reshaping industries and enhancing human capabilities across various sectors. In healthcare, ML algorithms are utilized for predictive analytics, diagnostic assistance, and personalized medicine, enabling practitioners to make more informed decisions based on large volumes of patient data. By analyzing historical health records and genetic information, AI can identify patterns that predict disease outbreaks or suggest tailored treatment plans, ultimately improving patient outcomes and reducing costs.
In finance, AI is used for fraud detection and algorithmic trading, allowing financial institutions to manage risks and optimize investments efficiently. Machine learning models analyze transaction patterns in real-time, identifying anomalies that may indicate fraudulent activity, thereby safeguarding assets and enhancing customer trust. Additionally, AI-driven trading algorithms can process vast amounts of market data at lightning speed, executing trades based on predictive analytics that human traders may overlook.
In the realm of transportation, AI facilitates advancements in autonomous vehicles, where machine learning algorithms interpret sensor data to navigate and operate safely. These intelligent systems enhance road safety and efficiency by minimizing human error and optimizing traffic flow. Moreover, AI revolutionizes supply chain management, enhancing logistics through demand forecasting and inventory optimization. By analyzing consumer behavior and market trends, AI can predict fluctuations in demand, enabling companies to adjust their inventory levels proactively and reduce waste.
Furthermore, the integration of AI in consumer technology has transformed the way individuals interact with devices. Virtual assistants, such as Amazon’s Alexa or Apple’s Siri, rely on natural language processing and machine learning to understand and respond to user queries, demonstrating the increasing prevalence of intelligent systems in everyday life. These advancements not only improve user experience but also pave the way for more sophisticated applications, such as smart home automation and personalized content delivery.
Beyond these sectors, AI and ML are making significant strides in education, where adaptive learning platforms tailor educational content to individual student needs, enhancing engagement and knowledge retention. In environmental science, AI models predict climate patterns and optimize resource management, contributing to sustainable practices.
As AI and ML continue to evolve, their potential to address complex challenges and enhance productivity across diverse industries is boundless. Embracing these technologies will be crucial for organizations aiming to remain competitive in an increasingly data-driven world.
Ethical Considerations in AI and Machine Learning
As AI and ML technologies continue to evolve, ethical considerations have become a central focus in debates surrounding their implementation. Concerns arise regarding privacy, bias, and accountability. For instance, algorithms trained on biased datasets can perpetuate and amplify existing biases, resulting in discriminatory outcomes in areas like hiring practices and law enforcement. Moreover, the use of AI in surveillance raises significant ethical questions around privacy and the extent to which individuals are monitored in public and private spaces.
The potential for AI to impact decision-making processes in critical sectors, such as healthcare and criminal justice, adds another layer of complexity to these ethical considerations. In healthcare, AI systems can assist in diagnosing diseases or recommending treatment plans; however, if these systems are not designed with equity in mind, they may inadvertently favor certain demographics over others, leading to health disparities. Similarly, in criminal justice, predictive policing algorithms can reinforce systemic biases, creating a cycle of over-policing in marginalized communities.
Transparency is another vital aspect of ethical AI development. Many AI algorithms operate as "black boxes," making it challenging for users and stakeholders to understand how decisions are made. This lack of transparency can hinder accountability, as individuals and organizations may struggle to challenge or appeal decisions made by AI systems. Therefore, developing explainable AI models that offer insights into their decision-making processes is essential to fostering trust and accountability.
Furthermore, the rapid advancement of AI technologies necessitates a proactive approach to ethical considerations. As innovation outpaces regulatory frameworks, there is a pressing need for stakeholders—including developers, policymakers, and ethicists—to collaborate in establishing comprehensive guidelines that govern AI development and implementation. These guidelines should address not only the technical aspects of AI but also the broader social implications, ensuring that technology serves the public good.
Future Challenges and Opportunities
The future of AI and ML presents both challenges and opportunities. One of the significant challenges lies in the need for transparency and explainability of algorithms. As these systems become increasingly embedded in critical decision-making processes, understanding how they arrive at specific conclusions is essential to build trust among users and stakeholders. The opacity of certain AI models can lead to unintended biases and ethical dilemmas, necessitating robust frameworks for accountability. Organizations must prioritize the development of interpretable models that not only yield accurate results but also provide insights into their decision-making processes.
Additionally, the rapid evolution of AI technologies necessitates a workforce that is equipped with relevant skills and knowledge. Educational institutions must adapt to this changing landscape by embedding AI and ML concepts into their curricula to prepare future generations for careers in these fields. This involves fostering interdisciplinary approaches that combine technical skills with ethical considerations, ensuring that graduates are not only proficient in programming and data analysis but also understand the societal implications of their work. Partnerships between academia and industry can facilitate internships and hands-on experiences, bridging the gap between theoretical knowledge and practical application.
On the other hand, the integration of AI and ML presents unique opportunities for innovation across various sectors. From improving healthcare outcomes through predictive analytics to enhancing efficiency in manufacturing with smart automation, AI technologies have the potential to drive economic growth and solve complex global challenges. For instance, in agriculture, machine learning algorithms can optimize crop yields by analyzing environmental data, ultimately contributing to food security. Furthermore, AI can play a pivotal role in combating climate change through optimized resource management, enabling organizations to reduce waste and enhance sustainability efforts.
Moreover, the proliferation of AI-driven solutions can lead to the creation of new markets and job opportunities, particularly in areas such as AI ethics, data privacy, and algorithm auditing. As businesses increasingly rely on AI tools, there will be a heightened demand for professionals who can navigate the complexities of these technologies while ensuring compliance with regulatory standards.
Conclusion
Artificial intelligence and machine learning represent transformative technologies that are reshaping various aspects of society. By understanding their foundations and applications, stakeholders can harness the potential of AI and ML while addressing ethical considerations that emerge from their implementation. As we move into the future, an emphasis on responsible development and deployment will be crucial to ensuring that AI and ML technologies positively impact individuals and society as a whole. Embracing these technologies promises not only to enhance operational efficiencies but also to unlock new avenues for innovation that can shape the trajectory of human progress.
What's Your Reaction?
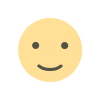
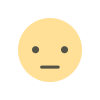
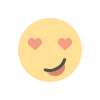
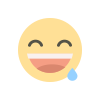
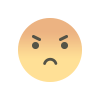
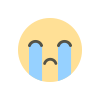
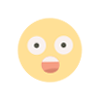