Data Analytics and Big data
Explore the world of Data Analytics and Big Data in our comprehensive guide. Learn how businesses leverage data to drive decisions, improve performance, and gain a competitive edge. Perfect for data professionals, business leaders, and tech enthusiasts!
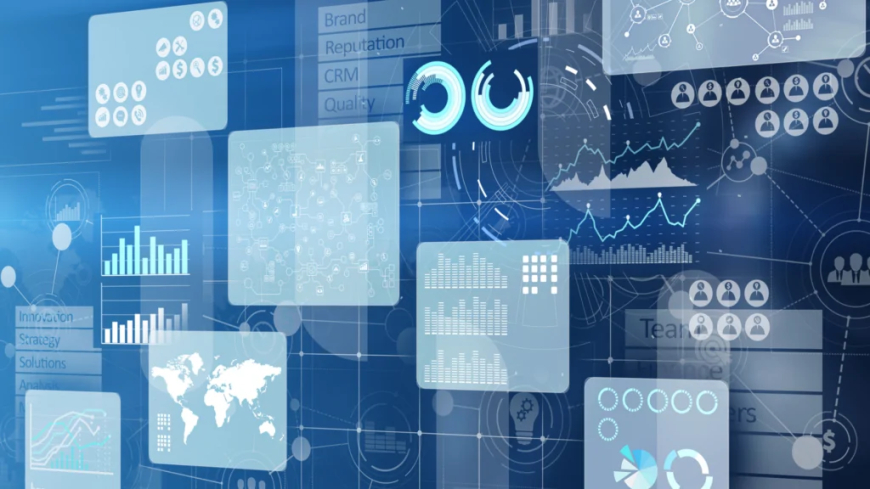
The terms "data analytics" and "big data" have gained significant attention; however, it is essential to understand their distinct meanings and implications. Both concepts pertain to the handling and interpretation of extensive data sets, yet they emphasize different aspects of this process.
Data analytics is the systematic examination of data to identify patterns, trends, and insights that can inform strategic business decisions. This process includes the collection, cleaning, and analysis of data to extract meaningful information. Techniques employed in data analytics may encompass statistical analysis, data mining, predictive modeling, and machine learning.
Conversely, big data refers to the unprecedented volume of data generated and collected at an extraordinary pace. It is characterized by three key attributes: volume, variety, and velocity. Volume pertains to the substantial amount of data produced, often measured in terabytes or petabytes. Variety encompasses the diverse forms of data collected, including text, images, videos, and social media content. Velocity indicates the rapid speed at which data is generated and the necessity for timely processing.
The challenges posed by big data are substantial, particularly in the realms of storage, processing, and analysis. Conventional data processing tools frequently fall short when confronted with such large and heterogeneous data sets. As a result, innovative technologies and methodologies, such as distributed computing systems and cloud computing, have emerged to tackle these challenges effectively.
The synergy between data analytics and big data holds remarkable potential for organizations across various sectors. By leveraging big data analytics, businesses can derive critical insights into customer behavior, market dynamics, and operational performance. These insights facilitate enhanced operational efficiency, informed decision-making, and the development of new products and services.
While data analytics and big data are interrelated, they serve different purposes. Data analytics focuses on extracting valuable insights from data, whereas big data pertains to the extensive quantities of data being produced. The integration of these two domains has the capacity to transform business operations and decision-making processes significantly.
Components of Data analysis and Big data:
When it comes to understanding the components of these concepts, there are a few key elements to consider.
1. Data: The foundation of big data and data analytics is the vast amount of information available. This data can come from various sources such as social media, sensors, web logs, and more. It is important to have relevant and good quality data for accurate analysis.
2. Data storage and management: With such large volumes of data, it is crucial to have efficient and scalable storage systems. Big data solutions often employ distributed file systems like Hadoop Distributed File System (HDFS) or cloud storage services to handle the data storage requirements. Data management also involves organizing and categorizing data to make it easily accessible for analysis.
3. Data processing and analysis: This component involves processing and analyzing the data to derive meaningful insights and patterns. This can be done through various statistical techniques, machine learning algorithms, or other data processing methods. The aim is to extract useful information from the data and make data-driven decisions.
4. Visualization: Once the data is analyzed, it needs to be presented in a way that is easily understandable and visually appealing. Data visualization tools and techniques help in representing the analyzed data in the form of charts, graphs, dashboards, and other visual formats. Visualization enhances the overall comprehension of the insights gained from the data.
5. Predictive analytics: This component involves using historical data to make predictions about future trends or outcomes. Predictive models are built using advanced algorithms to identify patterns, correlations, and make accurate predictions. This enables businesses to make proactive decisions and plan for the future based on data-driven insights.
6. Data security: Given the sensitivity of data, ensuring its security and protection is paramount. Data protection mechanisms such as encryption, access controls, and data governance frameworks are essential to safeguard the data from unauthorized access, loss, or theft. Compliance with regulations and privacy laws is also crucial in data analytics and big data.
7. Business intelligence: The final component involves using the insights gained from data analytics to drive business strategies and decision-making. By leveraging the power of big data and analytics, businesses can optimize operations, improve customer experience, identify new opportunities, and gain a competitive edge in the market.
Types and categories of data analysis:
There are various types and categories of data analysis that organizations can utilize to extract valuable information from the vast amount of data available. Below are some of the main types and categories of data analysis and big data:
1. Descriptive Analysis: Descriptive analysis focuses on summarizing and presenting the data in a meaningful way. It involves techniques such as data visualization, summarization, and aggregation to provide a clear understanding of the data's key features.
2. Diagnostic Analysis: Diagnostic analysis aims to determine why certain events occurred by analyzing past data. It involves examining patterns, relationships, and correlations to identify the root causes of specific outcomes or behaviors.
3. Predictive Analysis: Predictive analysis uses historical data to create models and make predictions about future outcomes. This type of analysis involves techniques such as regression analysis, time series analysis, and machine learning algorithms to forecast trends and probabilities.
4. Prescriptive Analysis: Prescriptive analysis goes a step further than predictive analysis by recommending actions or interventions to optimize future outcomes. It incorporates optimization techniques and algorithms to identify the best course of action based on the given data.
5. Exploratory Analysis: Exploratory analysis is an open-ended approach to data analysis, allowing analysts to examine data from different angles and discover new patterns or insights. This type of analysis is often used to identify trends, outliers, or anomalies that may not be immediately apparent.
6. Text Analysis: Text analysis involves extracting information and insights from unstructured text data such as emails, social media posts, customer reviews, or surveys. Natural language processing techniques and sentiment analysis are commonly used to analyze textual data.
7. Social Network Analysis: Social network analysis examines relationships and interactions between individuals or entities within a network. It helps to identify influential individuals, key connections, and the overall structure of a network.
When it comes to big data, the volume, velocity, and variety of data involved require specific approaches to analysis. Big data analysis often involves technologies like Hadoop, Spark, and data mining techniques to handle and extract insights from large datasets.
Common Problem with Data analysis and Big Data:
There are certain challenges that organizations often come across when dealing with data analysis and big data. Let me outline and discuss some of the common issues and their potential solutions.
1. Data quality: One of the major problems in data analysis is poor data quality. This can be due to errors, inconsistencies, or missing values in the dataset. To overcome this issue, organizations should invest in data cleansing and validation processes. By removing duplicate records, correcting errors, and filling in missing data, they can significantly improve data quality.
2. Data integration: Organizations often have data stored in multiple systems and formats, which makes data integration a challenging task. This problem can be addressed by using data integration tools that enable seamless merging of data from different sources. These tools help in creating a unified view of the data, making it easier to analyze and derive insights.
3. Scalability: Big data analysis involves processing vast amounts of data, which can strain the capacity of traditional data analysis tools. To overcome scalability issues, organizations should consider using big data platforms like Hadoop or Apache Spark. These platforms are designed to handle large-scale data processing and can effectively handle the volume, velocity, and variety of big data.
4. Data security and privacy: With the increasing amount of data being collected, organizations need to ensure data security and privacy. Encryption techniques and access controls can be implemented to protect sensitive data. Additionally, organizations should comply with data protection regulations to maintain the trust of their customers.
5. Lack of skilled professionals: Data analysis requires individuals with strong analytical and technical skills. However, there is a shortage of skilled data analysts and data scientists. To address this issue, organizations can invest in training programs and workshops to enhance the skills of their existing workforce. Collaboration with educational institutions can also help in fostering a new generation of data professionals.
6. Interpretation of results: Analyzing big data can provide valuable insights. However, interpreting these results and translating them into actionable strategies can be a complex task. To overcome this challenge, organizations should focus on data visualization techniques and data storytelling. These approaches can help in presenting data in a meaningful and easily understandable format, facilitating decision-making.
What's Your Reaction?
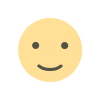
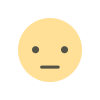
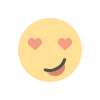
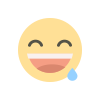
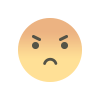
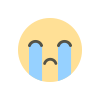
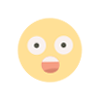