Data Analytics and Big Data: Transforming Decision-Making in the Digital Age
Discover how data analytics and big data are transforming decision-making in the digital age. Learn about predictive analytics, data visualization, and the impact of AI on modern business strategies.
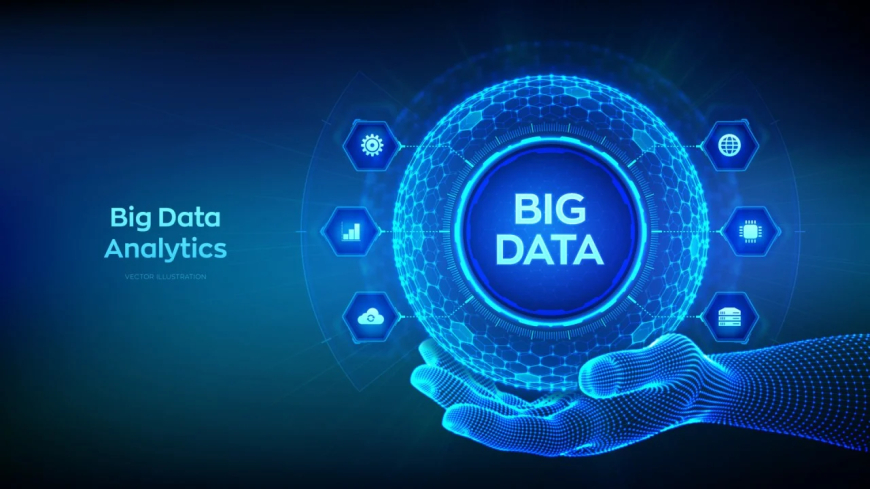
Introduction
In today's digital landscape, data has become the lifeblood of organizations across various sectors. With the proliferation of digital technologies, businesses generate and collect unprecedented volumes of data. This monumental shift has given rise to the concepts of Big Data and Data Analytics, which are now essential tools for decision-making processes. By enabling organizations to harness and interpret vast amounts of information, these technologies are fundamentally transforming how businesses operate, strategize, and compete.
Big Data refers to the enormous datasets that are so complex and voluminous that traditional data processing applications are inadequate to handle them. It encompasses various types of data, including structured, semi-structured, and unstructured data, which can be generated from numerous sources such as social media, IoT devices, and transactional systems. Data Analytics, on the other hand, is the process of examining these datasets to uncover patterns, correlations, and insights that can inform strategic decisions. Together, they create a powerful synergy that allows organizations to make data-driven choices that enhance operational efficiency and drive innovation.
The applications of Big Data and Data Analytics are vast and varied, spanning industries such as healthcare, finance, retail, and transportation. In healthcare, for instance, analytics can predict patient outcomes, optimize treatment plans, and improve operational efficiency. In finance, it enables risk assessment and fraud detection, while in retail, it enhances customer experience through personalized marketing strategies. Transportation companies leverage these technologies to optimize routes and manage logistics, ultimately reducing costs and improving service delivery.
However, the adoption of Big Data and Data Analytics is not without its challenges. Organizations often face issues related to data quality, privacy concerns, and the need for skilled personnel capable of interpreting complex datasets. Additionally, integrating these technologies into existing systems can be a daunting task, requiring significant investment in infrastructure and training.
Despite these challenges, the potential of Big Data and Data Analytics to influence decision-making in the digital age is immense. As organizations continue to embrace these technologies, they will unlock new opportunities for growth, innovation, and competitive advantage. The ability to turn data into actionable insights will not only enhance operational efficiency but also foster a culture of informed decision-making that is crucial for success in an increasingly data-driven world. In conclusion, as we navigate this evolving digital landscape, the mastery of Big Data and Data Analytics will be pivotal in shaping the future of business.
Understanding Big Data and Data Analytics
- Defining Big Data
Big Data refers to data sets that are so large, fast, or complex that traditional data processing methods are inadequate to handle them. Big Data is commonly characterized by the "Three Vs":
-
Volume: The sheer amount of data generated daily, which often reaches terabytes or petabytes. This explosion of data is driven by various sources, including social media interactions, IoT devices, and transactional records.
-
Velocity: The speed at which data is generated, collected, and processed, requiring real-time or near-real-time analytics. Organizations must be equipped to respond swiftly to changing data landscapes, ensuring they remain competitive and informed.
-
Variety: The different types of data sources, including structured data (relational databases), unstructured data (text, audio, video), and semi-structured data (XML, JSON). This diversity necessitates sophisticated data integration techniques to derive meaningful insights.
In this context, Big Data encompasses both the challenges and opportunities presented by this expansive data landscape. Organizations that harness Big Data effectively can unlock transformative insights that drive innovation and improve operational efficiencies.
- Data Analytics
Data Analytics involves the systematic computational analysis of data to uncover patterns, trends, correlations, and derive meaningful insights. There are several levels of data analytics:
-
Descriptive Analytics: This type involves understanding historical data and events through reporting tools. It answers the question, "What happened?" By summarizing past events, organizations can identify key performance indicators and establish benchmarks.
-
Diagnostic Analytics: This goes a step further, investigating the causes behind trends and events. It answers, "Why did this happen?" By employing techniques such as root cause analysis, organizations can refine their strategies and address underlying issues.
-
Predictive Analytics: Utilizing statistical models and machine learning algorithms, predictive analytics forecasts future outcomes based on historical data. It addresses "What could happen?" This forward-looking approach enables organizations to proactively manage risks and seize opportunities.
-
Prescriptive Analytics: This advanced level provides recommendations for actions based on predictive insights, effectively answering, "What should we do?" By simulating various scenarios, prescriptive analytics empowers decision-makers to choose the best course of action.
Together, Big Data and Data Analytics facilitate data-driven decision-making, where insights gleaned from analytics inform strategies, actions, and policies. As organizations continue to navigate the complexities of the digital age, the integration of Big Data and analytics will be pivotal in fostering a culture of innovation and resilience. Embracing these tools not only enhances operational performance but also positions organizations to thrive in an increasingly data-centric world.
Applications of Big Data and Data Analytics
The transformative potential of Big Data and analytics is evident across several industries. Below are some key applications:
-
Healthcare In healthcare, big data and analytics have revolutionized patient care and operational efficiency. Electronic health records (EHRs), wearables, and genomic data yield vast information that enables healthcare providers to improve outcomes. Predictive analytics model patient outcomes, while tracking and analysis of treatment efficacy informs personalized medicine strategies. Moreover, big data aids in epidemic surveillance and resource allocation in response to public health crises. For instance, during the COVID-19 pandemic, real-time data analytics facilitated contact tracing and vaccine distribution, showcasing the critical role of data in public health initiatives.
-
Retail In the retail sector, data analytics allows organizations to understand consumer behavior better, manage inventory effectively, and optimize pricing strategies. By analyzing transaction data, customer reviews, and social media interactions, retailers can enhance marketing campaigns and personalize customer experiences, ultimately boosting sales and customer loyalty. Advanced analytics tools enable businesses to forecast trends and customer preferences, allowing them to stay ahead of the competition and adapt swiftly to market changes.
-
Financial Services Big data and analytics play a critical role in risk management, fraud detection, and compliance in the financial services industry. Financial institutions leverage predictive analytics to assess credit risk and identify unusual patterns that may signal fraudulent activities. Furthermore, real-time data analysis aids traders in making well-informed investment decisions. The use of machine learning algorithms also enhances portfolio management, enabling firms to optimize asset allocation based on market conditions and investor behavior.
-
Marketing In marketing, data analytics is essential for understanding target audiences and measuring the effectiveness of campaigns. By analyzing customer demographics, engagement metrics, and conversion rates, businesses can refine their marketing strategies, allocate resources more effectively, and optimize return on investment. Advanced segmentation techniques allow marketers to tailor their messages, ensuring that campaigns resonate with specific consumer segments, thereby increasing engagement and conversion rates.
-
Transportation and Logistics Big data has transformed logistics and supply chain management. Companies utilize data analytics to optimize routes, reduce delivery times, and minimize costs. By monitoring vehicle performance and analyzing traffic patterns, businesses can enhance operational efficiency and improve customer satisfaction. Predictive maintenance powered by data analytics further ensures that vehicles are serviced proactively, reducing downtime and operational disruptions.
The applications of big data and analytics span multiple sectors, driving innovation and efficiency. As organizations continue to harness these powerful tools, they unlock new opportunities for growth and improvement, ultimately shaping the future of industries worldwide.
Challenges in Implementing Big Data and Analytics
Despite the promising applications of Big Data and data analytics, organizations face several challenges when attempting to implement these technologies effectively.
-
Data Quality and Integrity High-quality data is fundamental to successful analytics. Inaccurate or incomplete data can lead to misleading insights and poor decision-making. Organizations must establish robust data governance frameworks to ensure data integrity and quality across all sources. This involves not only regular data audits but also cultivating a culture of accountability where employees understand the importance of data accuracy. A proactive approach to data cleansing and validation can help mitigate the risks associated with poor data quality.
-
Skills Gap The deployment of advanced data analytics requires skilled personnel with expertise in data science, statistics, and machine learning. However, there is a noticeable talent gap in this field, leading to challenges in finding qualified professionals capable of leveraging big data effectively. Organizations must invest in training and development programs to upskill existing employees while also exploring partnerships with educational institutions to create a pipeline of future talent. Additionally, adopting user-friendly analytics tools can empower non-technical staff to contribute to data-driven initiatives, thereby bridging the skills gap.
-
Data Privacy and Security The collection and analysis of large data volumes raise concerns regarding data privacy and security. Organizations must navigate regulations like the General Data Protection Regulation (GDPR) and ensure that sensitive data is handled responsibly to protect consumers and maintain compliance. This necessitates the implementation of robust cybersecurity measures and data encryption techniques to safeguard information from breaches. Furthermore, fostering transparency with customers about data usage can enhance trust and mitigate privacy concerns.
-
Infrastructure and Cost Investing in the necessary technology infrastructure to store, process, and analyze big data entails significant costs. Organizations need to weigh the financial implications of implementing big data solutions and consider leveraging cloud services to reduce capital expenditures. Cloud-based platforms offer scalability and flexibility, allowing organizations to adjust resources according to their needs without incurring hefty upfront investments. However, organizations must also evaluate the long-term costs associated with cloud services, including data transfer fees and potential vendor lock-in.
-
Integration of Diverse Data Sources A critical challenge lies in integrating diverse data sources, which may include structured, semi-structured, and unstructured data from various platforms. Organizations often struggle to create a unified view of their data, which can hinder comprehensive analysis. Implementing standardized data formats and utilizing advanced integration tools can facilitate smoother data consolidation processes. Additionally, fostering collaboration between IT and business units is essential for ensuring that data integration strategies align with organizational objectives.
While the potential of Big Data and analytics is immense, organizations must navigate these challenges with strategic foresight and a commitment to continuous improvement. By addressing data quality, bridging the skills gap, ensuring privacy and security, managing infrastructure costs, and integrating diverse data sources, organizations can unlock the full value of their data assets.
The Future of Data Analytics and Big Data
As technology continues to evolve, the future of data analytics and big data is likely to be shaped by several trends:
-
Artificial Intelligence Integration The integration of AI and machine learning with big data analytics enhances predictive capabilities and enables automation in data-driven decision-making. AI algorithms can analyze vast datasets more effectively than human analysts, leading to faster insights and improved accuracy. This synergy will not only streamline operations but also empower organizations to uncover patterns and correlations that were previously obscured, facilitating proactive strategies that can adapt to market changes.
-
Real-Time Analytics The demand for real-time analytics continues to grow as businesses seek immediate insights to drive quick decision-making. Technologies such as stream processing and in-memory databases allow organizations to analyze data as it is generated, enabling timely responses to emerging trends. This shift towards real-time analytics will be critical in sectors like finance, healthcare, and e-commerce, where split-second decisions can significantly impact outcomes. Companies that harness real-time data will gain a competitive edge, allowing them to anticipate customer needs and tailor their offerings dynamically.
-
Enhanced Visualization Tools Data visualization tools will advance, providing intuitive interfaces that make complex data sets more accessible. By simplifying analytics through interactive dashboards and visual representations, organizations can improve their ability to interpret and act upon data insights. The future will see the rise of augmented analytics, where advanced visualization integrates seamlessly with AI, allowing users to explore data interactively and derive insights without needing extensive technical expertise. This democratization of data will empower employees across all levels to make informed decisions.
-
Emphasis on Ethical Data Use As awareness of data privacy grows, organizations will increasingly prioritize ethical data practices. This includes transparent communication with consumers about data usage and the implementation of privacy-preserving techniques in data analytics. The establishment of robust ethical frameworks will not only build trust with consumers but also ensure compliance with evolving regulations. Companies that prioritize ethical data use will likely foster stronger relationships with their customers, enhancing brand loyalty and reputation.
-
Decentralized Data Management The future of data analytics will also witness a shift towards decentralized data management systems, such as blockchain technology. This approach enhances data security and integrity, allowing organizations to maintain control over their data while ensuring transparency. Decentralization will enable secure sharing of data across platforms and stakeholders, fostering collaboration while minimizing risks associated with data breaches.
The future of data analytics and big data is poised for transformative changes driven by technological advancements and evolving consumer expectations. Organizations that embrace these trends will not only enhance their analytical capabilities but also redefine their strategic approaches in an increasingly data-centric world.
Conclusion
Data analytics and big data are transforming decision-making in the digital age by providing organizations with the tools needed to harness the power of information. The ability to analyze large and diverse data sets leads to enhanced understanding, improved operational efficiency, and innovative strategies that drive competitive advantages. As organizations increasingly rely on data-driven insights, they are discovering that the integration of advanced analytics into their business processes is not merely a trend but a necessity.
In this evolving landscape, the potential of big data extends beyond mere operational enhancements. It fosters a culture of informed decision-making, enabling organizations to anticipate market trends, personalize customer experiences, and optimize resource allocation. By employing predictive analytics, businesses can forecast future outcomes with remarkable accuracy, allowing them to stay ahead of the competition. Moreover, the insights gleaned from big data analytics can inform product development and marketing strategies, ensuring that offerings align closely with customer needs and preferences.
However, the journey toward effective data utilization is not without its challenges. Issues such as data quality, skills gaps, and privacy concerns require ongoing attention and strategic planning. Organizations must invest in robust data governance frameworks to ensure the integrity and security of their data assets. Additionally, fostering a workforce skilled in data literacy is essential. By cultivating an environment where employees are equipped to interpret and utilize data, organizations can unlock the full potential of their analytics initiatives.
Looking ahead, the future of big data and analytics promises new opportunities for businesses willing to embrace these transformative technologies. The emergence of artificial intelligence and machine learning is set to revolutionize data processing capabilities, enabling organizations to derive deeper insights and automate decision-making processes. As these technologies continue to evolve, the ability to leverage data effectively will become increasingly vital for organizations seeking to thrive in an era characterized by rapid technological advancement and dynamic market conditions.
In conclusion, the integration of data analytics and big data into strategic planning is not just advantageous; it is imperative for survival in today’s competitive landscape. Organizations that harness these tools will not only enhance their operational efficiency but also position themselves as leaders in innovation and customer satisfaction, ultimately driving sustainable growth in an ever-changing world.